Parallélisme : travaux dirigés et pratiques
Table des matières
5. MPI
5.1. Introduction
MPI (The Message Passing Interface),
conçue en 1993-94, est une norme (ou API - Application Programming Interface) définissant une bibliothèque de fonctions,
utilisable avec les langages C, C++ et Fortran. Elle permet d'exploiter des ordinateurs distants ou multiprocesseurs
par passage de messages (Wikipedia).
La technique de passage de message consiste à transmettre au travers du réseau les données à échanger. Initialement MPI a été conçu pour des systèmes
à mémoire distribuée très populaires dans les années 80-90. MPI fut ensuite adapté pour les systèmes à mémoire partagée et fonctionne à présent pour
des systèmes hybrides (distribué + partagé).
L'ensemble des fonctions peut être trouvé à cette adresse OpenMPI Doc.
MPI étant une API, il existe plusieurs implantations come MPICH ou OpenMPI (voir ce site pour
un aperçu de l'architecture MPI).
5.2. Installation
Sous Ubuntu 24.04, il n'est plus possible d'utiliser la partie C++ de MPI. Deux possibilités s'offrent à vous :
- installer OpenMPI à partir des fichiers sources d'une ancienne version (4.1 par exemple)
- utiliser un archive docker qui utilise OpenMPI
5.2.1. Installation OpenMPI depuis le code source
Récupérez openmpi-4.1.5.tar.gz sur le site d'OpenMPI.
Décompressez, compilez le code source et installez les librairies :
tar -xvzf openmpi-4.1.5.tar.gz
cd openmpi-4.1.5/
./configure --prefix=/usr/local/openmpi-4.1 --enable-mpi-cxx --disable-cuda
make -j4
sudo make install
Ici l'option --disable-cuda est censée supprimer la compilation de la partie CUDA mais semble
ne pas fonctionner ou ne pas être reconnue en général.
Modifiez ensuite votre fichier .bashrc :
export PATH=/usr/local/openmpi-4.1/bin:\$PATH
export LD_LIBRARY_PATH=/usr/local/openmpi-4.1/lib:\$LD_LIBRARY_PATH
5.2.2. Utilisation de Docker
Commencez par installer Docker s'il n'est pas déjà installé :
sudo apt-get update
# ne pas oublier docker-buildx pour les versions récentes de docker
sudo apt-get install docker.io docker-buildx
sudo usermod -aG docker $USER
sudo systemctl start docker
Créez ensuite un répertoire dans lequel vous placez un fichier Dockerfile :
mkdir mpi
cd mpi
nano Dockerfile
Le fichier Dockerfile possède le contenu suivant. Il crée un utilisateur user et
install OpenMPI ainsi que deux éditeurs en mode texte : nano et fte (commande sfte).
# Utiliser Ubuntu 20.04 comme image de base
FROM ubuntu:20.04
# Empêcher les invites interactives lors de l'installation des paquets
ARG DEBIAN_FRONTEND=noninteractive
# Mettre à jour le système et installer les dépendances nécessaires
# Utilisation de fte pour avoir l'éditeur de texte sfte plus sympathique
# que nano
RUN apt-get update && apt-get install -y \
build-essential \
openmpi-bin \
openmpi-common \
libopenmpi-dev \
sudo \
nano fte fte-console fte-terminal \
&& rm -rf /var/lib/apt/lists/*
# Créer un utilisateur non-root 'user'
RUN useradd -m -s /bin/bash user \
&& echo "user ALL=(ALL) NOPASSWD:ALL" >> /etc/sudoers
# Passer à l'utilisateur 'user'
USER user
# Créer un répertoire de travail pour les programmes
WORKDIR /home/user
# Configurer les variables d'environnement MPI
ENV PATH="/usr/lib64/openmpi/bin:\$PATH" \
LD_LIBRARY_PATH="/usr/lib64/openmpi/lib:\$LD_LIBRARY_PATH"
# L'utilisateur 'user' peut maintenant compiler et exécuter des programmes MPI
# Vous pouvez ajouter vos fichiers ou configurations ici, par exemple :
# COPY . /home/user/
# Commande par défaut (exemple pour démarrer un shell interactif)
CMD ["/bin/bash"]
Compilez ensuite le Dockerfile afin de créer le container MPI :
sudo docker build -t mpi_cpp .
sudo docker run -it mpi_cpp
Ou bien si on veut mapper (mettre en correspondance) le répertoire /home/richer/exchange de l'utilisateur
richer sur la machine hôte vers le répertoire /home/user/exchange du container :
docker run -it --mount type=bind,source=/home/richer/exchange,target=/home/user/exchange mpi_cpp
5.3. Utiliser MPI
Il est nécessaire de modifier substantiellement son programme si on désire le paralléliser avec MPI car
on exécute le même programme sur plusieurs machines/coeurs différents.
MPI est disponible pour Fortran, C et C++ et également avec Python (pyMPI).
Pour pouvoir disposer de MPI sur sa machine il faut installer les librairies suivantes sous Ubuntu :
sudo apt-get install libopenmpi-dev openmpi-bin openmpi-doc
Pour C et C++ on inclura le fichier mpi.h
Pour débuguer il est recommandé d'installer MPE (Multi-Processing Environment), difficile à trouver, qui doit être compilé lors de l'installation de Python Anaconda. On réalisera alors l'édition de liens avec -llmpe -lmpe
5.4. Communicateur
Les opérations MPI portent sur des communicateurs qui sont en quelque sorte des ports de communication inter processus.
Le communicateur par défaut est COMM_WORLD qui comprend tous les processus actifs.
On peut créer de nouveaux communicateurs mais cela se révèle complexe.
Le modèle de communication est un modèle point à point basé sur deux opérations élémentaires send
et receive qui sont ensuite déclinés de plusieurs manières différentes.
- broadcast : envoi une ou plusieurs données d'un membre vers les autres membres du groupe
- gather : rassembler des données des autres membres vers un membre pour en faire un tableau
- scatter : découpage d'un tableau et envoi de chaque partie du tableau aux autres membres du groupe
- reduce : algorithme de réduction
- scan : algorithme de scan
Le schéma classique d'utilisation de MPI consiste à :
- initialiser les ressources MPI_Init
- obtenir le nombre de processus lancés MPI_Comm_size
- obtenir l'identifiant du processus courant MPI_Comm_rank
- exécuter le code séquentiel
- exécuter le code parallèle
- libérer les ressources MPI_Finalize
Voici deux exemples simples qui affichent un message avec un temps de latence (instruction sleep)
pour chaque thread / processeur. Le premier utilise des fonctions C, le second utilise des espaces de noms et des objets
// ==================================================================
// Author: Jean-Michel Richer
// Email: jean-michel.richer@univ-angers.fr
// Date: Aug 2016
// Purpose: Demonstrate basic functionnalities of MPI with C
// ==================================================================
#include <iostream>
#include <cstdlib>
#include <unistd.h> // for sleep
using namespace std;
#include <mpi.h>
// ==============================================================
// C version
// ==============================================================
int main(int argc, char ** argv) {
int max_cpus, cpu_rank;
char cpu_name[MPI_MAX_PROCESSOR_NAME];
int length;
// allocate resources
MPI_Init(&argc, &argv);
// get number of programs running
MPI_Comm_size(MPI_COMM_WORLD, &max_cpus);
// get program identifier
MPI_Comm_rank(MPI_COMM_WORLD, &cpu_rank);
// get cpu name
MPI_Get_processor_name(cpu_name, &length);
sleep(cpu_rank);
cerr << "running on " << cpu_name << " with id=" << cpu_rank << "/";
cerr << max_cpus << endl;
// free resources
MPI_Finalize();
exit(EXIT_SUCCESS);
}
// ==================================================================
// Author: Jean-Michel Richer
// Email: jean-michel.richer@univ-angers.fr
// Date: Aug 2016
// Purpose: Demonstrate basic functionnalities of MPI with C++
// ==================================================================
#include <iostream>
#include <cstdlib>
#include <unistd.h> // for sleep
using namespace std;
#include <mpi.h>
// ==================================================================
// C++ version
// ==================================================================
int main(int argc, char ** argv) {
int max_cpus, cpu_rank;
char cpu_name[MPI::MAX_PROCESSOR_NAME];
int length;
// allocate resources
MPI::Init(argc, argv);
// get number of programs running
max_cpus = MPI::COMM_WORLD.Get_size();
// get program identifier
cpu_rank = MPI::COMM_WORLD.Get_rank();
// get cpu name
memset(cpu_name, 0, MPI::MAX_PROCESSOR_NAME);
MPI::Get_processor_name(cpu_name, length);
// sleep
sleep(cpu_rank);
cerr << "running on " << cpu_name << " with id=" << cpu_rank << "/";
cerr << max_cpus << endl;
// free resources
MPI::Finalize();
exit(EXIT_SUCCESS);
}
5.4.1. Compilation
Afin de compiler un programme MPI, on utilise le compilateur mpicc pour le C, mpic++ pour le C++ :
mpic++ -o exe src.cpp -O2 ...
5.4.2. Execution
Pour exécuter un programme compilé avec MPI, il faut utiliser mpirun (ou
mpiexec) en spécifiant le nombre de processeurs (=processus,coeurs) grâce à l'option -n ou -np suivant les systèmes :
mpirun -n 4 ./c3_mpi_ex_1.exe
Voici le résultat à l'affichage du programme précédent si on l'exécute sur une seule machine Intel Core i3-2375M CPU @ 1.50GHz
(Dual Core + HyperThreading, soit 4 threads) :
running on inspiron with id=0/4
running on inspiron with id=1/4
running on inspiron with id=2/4
running on inspiron with id=3/4
Voici un autre exemple qui lance 16 processus sur 4 noeuds en réservant 4 processus (coeurs) sur chacune des machines :
mpiexec -hosts h1:4,h2:4,h3:4,h4:4 -n 16 ./test
Sur un cluster on utilisera par exemple (-pe = parallel environment):
qsub -pe mpi 16 test_mpi.sh
5.5. Synchronisation
Pour obtenir une section critique pour l'affichage on utilise la fonction MPI_Barrier ou
MPI::COMM_WORLD.Barrier() en C++ qui bloque l'appelant jusqu'à ce que tous les autres programmes
aient appelé cette fonction :
// ==================================================================
// Author: Jean-Michel Richer
// Email: jean-michel.richer@univ-angers.fr
// Date: Aug 2016
// Purpose: Demonstrate basic functionalities of MPI with C++
// with synchronization for display
// ==================================================================
#include <iostream>
#include <cstdlib>
#include <unistd.h> // for sleep
using namespace std;
#include <mpi.h>
// ==================================================================
// C++ version
// ==================================================================
int main(int argc, char ** argv) {
int max_cpus, cpu_rank;
char cpu_name[MPI::MAX_PROCESSOR_NAME];
int length;
// allocate resources
MPI::Init(argc, argv);
// get number of programs running
max_cpus = MPI::COMM_WORLD.Get_size();
// get program identifier
cpu_rank = MPI::COMM_WORLD.Get_rank();
// get cpu name
memset(cpu_name, 0, MPI::MAX_PROCESSOR_NAME);
MPI::Get_processor_name(cpu_name, length);
cout << "Hello, from " << cpu_rank << endl;
sleep( cpu_rank + 1 );
MPI::COMM_WORLD.Barrier();
cout << "Bye, from " << cpu_rank << endl;
// free resources
MPI::Finalize();
exit(EXIT_SUCCESS);
}
Dans l'exemple qui suit, les 6 processus commencent par travailler (travail remplacé
ici par un sleep) en parallèle.
Le premier (identifiant 0) suspend son exécution pendant 1 seconde, le second pendant 2 secondes, etc.
Au final, après 6 secondes, l'ensemble des processus exécutent le code qui suit
l'appel à MPI::COMM_WORLD.Barrier();.
mpirun -n 6 ./mpi_cpp_syncrho.exe
Hello, from 3
Hello, from 4
Hello, from 5
Hello, from 2
Hello, from 0
Hello, from 1
.... wait 6 seconds here until you finally get ....
Bye, from 0
Bye, from 5
Bye, from 3
Bye, from 4
Bye, from 1
Bye, from 2
5.6. Echange de donneés Send, Recv
Pour transmettre des données entre les processeurs, on utilise deux fonctions MPI_Send et MPI_Recv, ou éventuellement
MPI_Sendrecv :
// envoi d'un processus vers un autre processus
int MPI_Send(void* data,
int count,
MPI_Datatype datatype,
int destination,
int tag,
MPI_Comm communicator)
// réception d'un processus vers un autre processus
int MPI_Recv(void* data,
int count,
MPI_Datatype datatype,
int source,
int tag,
MPI_Comm communicator,
MPI_Status* status)
// envoi suivi d'une réception
int MPI_Sendrecv(const void *sendbuf,
int sendcount, MPI_Datatype sendtype,
int dest, int sendtag,
void *recvbuf, int recvcount, MPI_Datatype recvtype,
int source, int recvtag,
MPI_Comm comm, MPI_Status *status)
- data
- pointeur sur la donnée à envoyer ou recevoir
- count
- nombre d'occurrences
- datatype
- type de donnée (MPI_CHAR, MPI_INT, MPI_FLOAT, MPI::INT, MPI::FLOAT, ...)
- source/destination
- identifiant du processus qui reçoit ou envoie les données
- communicator
- canal de comunication, ex: COMM_WORLD
- tag
- identifiant de message compris entre 0 et 32767
- status
- le status lors de la réception des données
Pour la partie C++ on utilisera :
MPI::COMM_WORLD.Send(const void* buf,
int count,
MPI::Datatype& datatype,
int dest,
int tag) const
void MPI::COMM_WORLD.Recv(void* buf,
int count,
MPI::Datatype& datatype,
int source,
int tag,
MPI::Status* status) const
void MPI::COMM_WORLD::Sendrecv(const void* sendbuf,
int count,
MPI::Datatype& datatype,
int dest, int sendtag,
void* recvbuf, int recvcount,
MPI::Datatype recvtype, int source, int recvtag,
MPI::Status* status) const
Voici quelques exemples :
Dans le premier exemple, on utilise deux processeurs. Le maître (identifiant 0) et l'esclave (identifiant 1) :
Création d'un tableau et initialisation |
|
Envoi de la taille → |
|
|
Réception de la taille, création du tableau |
Envoi du tableau → |
|
|
Réception des données dans le tableau |
|
Calcul de la somme des éléments |
|
← Envoi de la somme |
Réception de la somme |
|
Affichage de la somme |
|
Fonctionnement Exemple Send et Receive
// ==================================================================
// Author: Jean-Michel Richer
// Email: jean-michel.richer@univ-angers.fr
// Date: Aug 2016
// Purpose: Demonstrate basic send functionality, send array of float
// from master (rank=0) to slave (rank=1)
// ==================================================================
#include <unistd.h>
#include <iostream>
#include <cstdlib>
#include <unistd.h> // for sleep
using namespace std;
#include <mpi.h>
// ==============================================================
// C++ version
// ==============================================================
int main(int argc, char ** argv) {
int max_cpus, cpu_rank, remote_cpu;
char cpu_name[MPI::MAX_PROCESSOR_NAME];
int length;
// data to send
float *data;
int data_size;
// Initialize
MPI::Init(argc, argv);
max_cpus = MPI::COMM_WORLD.Get_size();
cpu_rank = MPI::COMM_WORLD.Get_rank();
MPI::Status status;
memset(cpu_name, 0, MPI::MAX_PROCESSOR_NAME);
MPI::Get_processor_name(cpu_name,length);
cerr << cpu_rank << "/" << max_cpus << " on machine " << cpu_name << endl;
// MASTER
if (cpu_rank == 0) {
// MASTER
// processor of id 0 (master) fills and sends the array
data_size = 100 + rand() % 100;
data = new float [data_size];
for (int i=0; i<data_size; ++i) data[i] = i+1;
int remote_cpu = 1;
// 1- send length
cerr << cpu_rank << " [send] data_size=" << data_size << endl;
MPI::COMM_WORLD.Send(&data_size, 1, MPI::INT, remote_cpu, 0);
// 2- send data
MPI::COMM_WORLD.Send(&data[0], data_size, MPI::FLOAT, remote_cpu, 0);
// wait for other processor to compute and send back sum
float result = 0;
MPI::COMM_WORLD.Recv(&result, 1, MPI::FLOAT, remote_cpu, MPI::ANY_TAG, status);
cerr << cpu_rank << " [recv] result=" << result << endl;
cerr << "result is " << result << " for length=" << data_size;
cerr << ", expected=" << (data_size * (data_size+1))/ 2 << endl;
delete [] data;
} else if (cpu_rank == 1) {
// SLAVE
remote_cpu = 0;
// processor of id 1 will receive the array and compute the sum
// 1- we receive the array length and allocate space
MPI::COMM_WORLD.Recv(&data_size, 1, MPI::INT, remote_cpu, MPI::ANY_TAG, status);
cerr << cpu_rank << " [recv] data_size=" << data_size << endl;
data = new float[data_size];
// 2- we receive the data
MPI::COMM_WORLD.Recv(&data[0], data_size, MPI::FLOAT, remote_cpu, MPI::ANY_TAG, status);
// 3- compute sum
float sum = 0;
for (int i=0; i<data_size; ++i) {
sum += data[i];
}
// 4- send back result
cerr << cpu_rank << " [send] sum=" << sum << endl;
MPI::COMM_WORLD.Send(&sum, 1, MPI::FLOAT, remote_cpu, 0);
delete [] data;
} else {
// other processors (if any) won't do anything
}
MPI::Finalize();
exit(EXIT_SUCCESS);
}
Le deuxième exemple utilise sendrecv pour envoyer les données du tableau et attendre de recevoir la somme.
#include <unistd.h>
#include <iostream>
#include <cstdlib>
#include <unistd.h> // for sleep
#include <sstream>
using namespace std;
#include <mpi.h>
int max_cpus, cpu_rank, remote_cpu;
char cpu_name[MPI::MAX_PROCESSOR_NAME];
ostringstream output;
void message() {
cout << "cpu " << cpu_rank << "/" << max_cpus;
cout << " on " << cpu_name << ": ";
cout << output.str() << endl;
cout.flush();
output.str("");
}
// ==============================================================
// version C++
// ==============================================================
int main(int argc, char ** argv) {
int length;
// data to send
float *data;
int data_length;
MPI::Init(argc, argv);
max_cpus = MPI::COMM_WORLD.Get_size();
cpu_rank = MPI::COMM_WORLD.Get_rank();
MPI::Status status;
memset(cpu_name, 0, MPI::MAX_PROCESSOR_NAME);
MPI::Get_processor_name(cpu_name,length);
message();
if (cpu_rank == 0) {
// processor of id 0 is filling and sending the array
data_length = 100 + rand() % 100;
data = new float [data_length];
for (int i=0; i<data_length; ++i) data[i] = i+1;
int remote_cpu = 1;
// 1- send length
output << "send data_length=" << data_length;
message();
MPI::COMM_WORLD.Send(&data_length, 1, MPI::INT, remote_cpu, 0);
// 2- send data wait for other processor to compute and send back sum
float result = 0;
MPI::COMM_WORLD.Sendrecv(
&data[0], data_length, MPI::FLOAT, remote_cpu, 0,
&result, 1, MPI::FLOAT, cpu_rank, MPI::ANY_TAG,
status);
output << "result is " << result << " for length=" << data_length;
message();
output << "result expected=" << (data_length * (data_length+1))/ 2 << endl;
message();
delete [] data;
} else if (cpu_rank == 1) {
// processor of id 1 will receive the array and compute the sum
// 1- we receive the array length and allocate space
remote_cpu = 0;
MPI::COMM_WORLD.Recv(&data_length, 1, MPI::INT, remote_cpu, MPI::ANY_TAG, status);
output << "receive data_length=" << data_length;
message();
data = new float[data_length];
// 2- we receive the data
MPI::COMM_WORLD.Recv(&data[0], data_length, MPI::FLOAT, remote_cpu, MPI::ANY_TAG, status);
// 3- compute sum
float sum = 0;
for (int i=0; i<data_length; ++i) {
sum += data[i];
}
// 4- send back result
MPI::COMM_WORLD.Send(&sum, 1, MPI::FLOAT, remote_cpu, 0);
delete [] data;
} else {
// other processors won't do anything
}
MPI::Finalize();
exit(EXIT_SUCCESS);
}
Afin de simplifier l'utilisation de MPI on peut créer une interface (wrapper) : j'ai créé, pour ma part, EZMPI.
EZMPI est constitué d'une seule classe Process qui représente un processus et qui permet de récupérer lors de l'initialisation
l'identifiant du processeur, le nombre de processus lancés, ...
On dispose également de méthodes qui permettent d'envoyer et recevoir un élément (quel que soit le type)
ou un tableau d'élements.
On dispose en outre d'un système de log qui permet de consulter le déroulement des échanges entre processus (réception et envoi de données).
// ==================================================================
// Author: Jean-Michel Richer
// Email: jean-michel.richer@univ-angers.fr
// Date: Aug 2020
// Last modified: November 2020
// Purpose: interface and class to facilitate use of MPI
// ==================================================================
#ifndef EZ_MPI_H
#define EZ_MPI_H
#include <string>
#include <typeinfo>
#include <sstream>
#include <stdexcept>
#include <cstdint>
using namespace std;
#include <time.h>
#include <unistd.h>
#include <mpi.h>
/**
* EZ MPI is a wrapper for MPI C++. It simplifies the use
* of MPI send, receive, gather, scatter functions.
* For the gather, scatter and reduce functions the processor
* of rank 0 is considered as the "master" that collects or
* sends data.
*/
namespace ez {
namespace mpi {
/*
* Process Information.
* This class also acts as a logger so it can be used to print
* information.
*/
class Process {
protected:
// maximum number of process working together
int m_max;
// identifier of current process, called rank for MPI
int m_id;
// identifier of remote process for send, receive, ...
int m_remote;
// status of last operation
MPI::Status m_status;
// message tag if needed (default is 0)
int m_message_tag;
// name of process
string m_name;
// Linux process identifier
int m_pid;
// verbose mode
bool m_verbose_flag;
// verbose mode
bool m_log_flag;
// main output stream for current processor
ostringstream log_stream;
// temporary output stream
ostringstream tmp_log;
private:
/**
* Find processor name
*/
void find_cpu_name();
/**
* record output of oss into general output
*/
void append();
/**
* initialize max_cpus, cpu_rank, processus id
*/
void init();
public:
/**
* Default constructor
*/
Process(int argc, char *argv[], bool verbose=false);
~Process();
/**
* Display output of each processor if verbose mode is on
*/
void finalize();
/**
* set verbose mode
*/
void verbose(bool mode) {
m_verbose_flag = mode;
}
/**
* set log mode
*/
void log(bool mode) {
m_log_flag = mode;
}
/**
* Get process identifier
*/
int pid();
/**
* Get processor identifier or rank
*/
int id();
/**
* Get number of processors used
*/
int max();
/**
* Get processor name
*/
string name();
/**
* set remote processor identifier
*/
void remote(int rmt);
/**
* set message tag
* @param tag must be an integer between 0 and 32767
*/
void tag(int tag);
/**
* Return true if this processor is the processor of rank 0
* considered as the master.
*/
bool is_master() {
return (m_id == 0);
}
/**
* synchronize
*/
void synchronize();
/**
* Determine type of data T and convert it into MPI::Datatype.
* This function needs to be extended with other types.
*/
template<class T>
MPI::Datatype get_type() {
if (typeid(T) == typeid(char)) {
return MPI::CHAR;
} else if (typeid(T) == typeid(int8_t)) {
return MPI::CHAR;
} else if (typeid(T) == typeid(uint8_t)) {
return MPI::CHAR;
} else if (typeid(T) == typeid(int)) {
return MPI::INT;
} else if (typeid(T) == typeid(float)) {
return MPI::FLOAT;
} else if (typeid(T) == typeid(double)) {
return MPI::DOUBLE;
}
//throw std::runtime_error("unknown MPI::Datatype");
cout << "!!!!! unknown " << typeid(T).name() << endl;
return MPI::INT;
}
/**
* Send one instance of data to remote_cpu
* @param v data to send
*/
template<class T>
void send(T& v) {
MPI::Datatype data_type = get_type<T>();
tmp_log << "send value=" << v << " to " << m_remote << endl;
flush();
MPI::COMM_WORLD.Send(&v, 1, data_type, m_remote, m_message_tag);
}
/**
* Send an array to remote_cpu
* @param arr address of the array
* @param size number of elements to send
*/
template<class T>
void send(T *arr, int size) {
MPI::Datatype data_type = get_type<T>();
tmp_log << "send array of size=" << size << " to " << m_remote << endl;
flush();
MPI::COMM_WORLD.Send(&arr[0], size, data_type, m_remote, m_message_tag);
}
/**
* Receive one instance of data from remote cpu
* @param v data to receive
*/
template<class T>
void recv(T& v) {
MPI::Datatype data_type = get_type<T>();
MPI::COMM_WORLD.Recv(&v, 1, data_type, m_remote,
(m_message_tag == 0) ? MPI::ANY_TAG : m_message_tag,
m_status);
tmp_log << "receive value=" << v << " from " << m_remote << endl;
flush();
}
/**
* Receive an array of given size
* @param arr pointer to address of the array
* @param size number of elements
*/
template<class T>
void recv(T *arr, int size) {
MPI::Datatype data_type = get_type<T>();
MPI::COMM_WORLD.Recv(&arr[0], size, data_type, m_remote,
(m_message_tag == 0) ? MPI::ANY_TAG : m_message_tag,
m_status);
tmp_log << "receive array of size=" << size << " from " << m_remote << endl;
flush();
}
/**
* Send array and receive value in return, this is an instance
* of the Sendrecv function.
* @param arr address of the array to send
* @param size size of the array to send
* @param value value to receive
*/
template<class T, class U>
void sendrecv(T *array, int size, U& value) {
MPI::Datatype array_data_type = get_type<T>();
MPI::Datatype value_data_type = get_type<U>();
tmp_log << "sendrecv/send array of size=" << size << endl;
flush();
MPI::COMM_WORLD.Sendrecv(&array[0], size, array_data_type, m_remote, 0,
&value, 1, value_data_type, MPI::ANY_SOURCE, MPI::ANY_TAG,
m_status);
tmp_log << "sendrecv/receive value=" << value << endl;
flush();
}
/**
* Perform reduction
* @param lcl_value local array used to perform reduction
* @param glb_value global data that will contain result
* @param op operation to perform (MPI::SUM, MPI::MAX, ...)
*/
template<class T>
void reduce(T &lcl_value, T &glb_value, const MPI::Op& op) {
MPI::Datatype data_type = get_type<T>();
MPI::COMM_WORLD.Reduce(&lcl_value,
&glb_value, 1, data_type, op, 0);
tmp_log << "reduction gives value=" << glb_value << endl;
flush();
}
/**
* Perform gather operation
* @param lcl_array local array that is send to master process
* @param glb_array global array that will contain all local arrays
*/
template<class T>
void gather(T *lcl_array, int size, T *glb_array) {
MPI::Datatype data_type = get_type<T>();
MPI::COMM_WORLD.Gather(lcl_array, size, data_type,
glb_array, size, data_type, 0);
tmp_log << "gather" << endl;
flush();
}
/**
* Perform scatter operation
* @param glb_array array of data that will be send by to all processors
* @param size size of the local array of data
* @param lcl_array local array of data
*/
template<class T>
void scatter(T *glb_array, int size, T *lcl_array) {
MPI::Datatype data_type = get_type<T>();
MPI::COMM_WORLD.Scatter(glb_array, size, data_type,
lcl_array, size, data_type, 0);
tmp_log << "scatter" << endl;
flush();
}
typedef std::ostream& (*ManipFn)(std::ostream&);
typedef std::ios_base& (*FlagsFn)(std::ios_base&);
void print(char v);
void print(int v);
void print(string s);
void print(float f);
void print(double d);
template<class T> // int, double, strings, etc
Process& operator<<(const T& output) {
tmp_log << output;
return *this;
}
// endl, flush, setw, setfill, etc.
Process& operator<<(ManipFn manip) {
manip(tmp_log);
if (manip == static_cast<ManipFn>(std::flush) || manip == static_cast<ManipFn>(std::endl)) {
this->flush();
}
return *this;
}
// setiosflags, resetiosflags
Process& operator<<(FlagsFn manip) {
manip(tmp_log);
return *this;
}
void flush();
void logs(ostream& out);
typedef void (*Code)(Process& p);
void run(Code code) {
code(*this);
}
};
} // end of namespace mpi
} // end of namespace ez
#endif
// ==================================================================
// Author: Jean-Michel Richer
// Email: jean-michel.richer@univ-angers.fr
// Date: Aug 2020
// Last modified: November 2020
// Purpose: interface and class to facilitate use of MPI
// ==================================================================
#include "ezmpi.h"
using namespace ez::mpi;
void Process::find_cpu_name() {
char name[MPI::MAX_PROCESSOR_NAME];
int length;
memset(name, 0, MPI::MAX_PROCESSOR_NAME);
MPI::Get_processor_name(name,length);
m_name = name;
}
void Process::init() {
m_max = MPI::COMM_WORLD.Get_size();
m_id = MPI::COMM_WORLD.Get_rank();
find_cpu_name();
m_pid = getpid();
if (m_verbose_flag) {
tmp_log << "pid=" << m_pid << ", id=" << m_id << endl;
flush();
}
}
Process::Process(int argc, char *argv[], bool verbose) {
m_remote = 0;
m_message_tag = 0;
m_verbose_flag = verbose;
m_log_flag = false;
MPI::Init(argc, argv);
init();
}
void Process::logs(ostream& out) {
m_verbose_flag = m_log_flag = false;
if (m_id == 0) {
out.flush();
out << std::endl;
out << "====================" << std::endl;
out << "=== FINAL RESULT ===" << std::endl;
out << "====================" << std::endl;
out << "---------------------" << std::endl;
out << "CPU " << m_id << std::endl;
out << "---------------------" << std::endl;
out << log_stream.str();
out.flush();
remote( 1 );
int token = -255;
send( token );
} else {
remote( m_id - 1 );
int token;
recv(token);
out << "---------------------" << std::endl;
out << "CPU " << m_id << std::endl;
out << "---------------------" << std::endl;
out << log_stream.str();
out.flush();
if (m_id < m_max - 1) {
remote( m_id + 1 );
token = -255;
send(token);
}
}
}
void Process::finalize() {
MPI::COMM_WORLD.Barrier();
MPI::Finalize();
}
Process::~Process() {
finalize();
}
int Process::pid() {
return m_pid;
}
int Process::id() {
return m_id;
}
int Process::max() {
return m_max;
}
string Process::name() {
return m_name;
}
void Process::tag(int tag) {
m_message_tag = tag;
}
void Process::remote(int rmt) {
m_remote = rmt;
}
void Process::synchronize() {
MPI::COMM_WORLD.Barrier();
}
// --------------------------
// output
// --------------------------
const std::string currentDateTime() {
time_t now = time(0);
struct tm tstruct;
char buf[80];
tstruct = *localtime(&now);
//strftime(buf, sizeof(buf), "%Y-%m-%d.%X [%s]", &tstruct);
strftime(buf, sizeof(buf), "%X", &tstruct);
return buf;
}
void Process::flush() {
string str = currentDateTime();
if (m_log_flag) {
log_stream << str << " cpu " << m_id << "/" << m_max << ": " << tmp_log.str();
}
if (m_verbose_flag) {
cerr << str << " cpu " << m_id << "/" << m_max << ": " << tmp_log.str();
}
tmp_log.str("");
}
void Process::print(char v) {
tmp_log << v;
}
void Process::print(int v) {
tmp_log << v;
}
void Process::print(string v) {
tmp_log << v;
}
void Process::print(float v) {
tmp_log << v;
}
void Process::print(double v) {
tmp_log << v;
}
On peut alors réécrire les programes précédents de manière plus simple :
// ==================================================================
// Author: Jean-Michel Richer
// Email: jean-michel.richer@univ-angers.fr
// Date: Aug 2020
// Last modified: November 2020
// Purpose: Demonstrate basic send functionality, send array of float
// from master (rank=0) to slave (rank=1)
// ==================================================================
#include <unistd.h>
#include <iostream>
#include <cstdlib>
#include <unistd.h> // for sleep
using namespace std;
#include "ezmpi.h"
using namespace ez::mpi;
/**
* run master and slaves
*/
void run(int argc, char *argv[]) {
// data to send
float *data;
int data_size;
// Create Process from ezmpi
// that retrives cpu rank (id), # cpus (max), cpu name (name)
Process p(argc, argv);
// the master is the process with id() == 0
if (p.is_master()) {
// Code of master
// set remote cpu identifier that will communicate with master
p.remote(1);
// processor of id 0 (master) fills and sends the array
data_size = 100 + rand() % 100;
data = new float [data_size];
for (int i=0; i<data_size; ++i) data[i] = i+1;
// 1- send size of array to remote
p.send(data_size);
// 2- send data of array to remote
p.send(data, data_size);
// wait for other processor to compute and send back sum
float result = 0;
p.recv(result);
p << "result is " << result << " for length=" << data_size;
p << ", expected=" << (data_size * (data_size+1))/ 2 << endl;
delete [] data;
} else if (p.id() == 1) {
// Code for slave
// tells to Process to send data to master processor
p.remote(0);
// process of id 1 will receive the array and compute the sum
// 1- we receive the array length and allocate space
p.recv(data_size);
data = new float[data_size];
// 2- we receive the data
p.recv(data, data_size);
// 3- compute sum
float sum = 0;
for (int i=0; i<data_size; ++i) {
sum += data[i];
}
// 4- send back result
p.send(sum);
delete [] data;
} else {
// other process (if any) won't do anything
p << "is idle" << endl;
}
sleep(1);
p.logs(cout);
// desctuctor of Process p will call the finalize method
// of MPI
}
/**
* main function
*
*/
int main(int argc, char ** argv) {
// call a function that contains code for master and slave
// in order to avoid problems of initialization and finalization
run(argc, argv);
exit(EXIT_SUCCESS);
}
// ==================================================================
// Author: Jean-Michel Richer
// Email: jean-michel.richer@univ-angers.fr
// Date: Aug 2020
// Last modified: November 2020
// Purpose: Demonstrate basic send functionality, send array of float
// from master (rank=0) to slave (rank=1)
// ==================================================================
#include <unistd.h>
#include <iostream>
#include <cstdlib>
#include <unistd.h> // for sleep
#include <sstream>
using namespace std;
#include "ezmpi.h"
using namespace ez::mpi;
/**
* run master and slaves
*/
void run(int argc, char *argv[]) {
// data to send
float *data;
int data_size;
// Create Process from ezmpi
// that retrives cpu rank (id), # cpus (max), cpu name (name)
Process p(argc, argv);
if (p.is_master()) {
// Code of master
// set remote cpu identifier that will communicate with master
p.remote(1);
// processor of id 0 (master) fills and sends the array
data_size = 100 + rand() % 100;
data = new float [data_size];
for (int i=0; i<data_size; ++i) data[i] = i+1;
// 1- send size of array to remote
p.send(data_size);
// 2- send data of array to remote and receive result
float result = 0;
p.sendrecv(data, data_size, result);
p << "result is " << result << " for length=" << data_size;
p << ", expected=" << (data_size * (data_size+1))/ 2 << endl;
delete [] data;
} else if (p.id() == 1) {
// Code for slave
// tells to Process to send data to master processor
p.remote(0);
// process of id 1 will receive the array and compute the sum
// 1- we receive the array length and allocate space
p.recv(data_size);
data = new float[data_size];
// 2- we receive the data
p.recv(data, data_size);
// 3- compute sum
float sum = 0;
for (int i=0; i<data_size; ++i) {
sum += data[i];
}
// 4- send back result
p.send(sum);
delete [] data;
} else {
// other process (if any) won't do anything
p << "is idle" << endl;
}
sleep(1);
p.logs(cout);
// desctuctor of Process p will call the finalize method
// of MPI
}
// ==============================================================
// version C++
// ==============================================================
int main(int argc, char ** argv) {
// call a function that contains code for master and slave
// in order to avoid problems of initialization and finalization
run(argc, argv);
exit(EXIT_SUCCESS);
}
5.7. Réduction
Certains traitements comme la réduction ou le scan sont implantés spécifiquement pour MPI.
Par exemple pour la réduction :
// ==================================================================
// Author: Jean-Michel Richer
// Date: 20 Aug 2016
// Purpose: Use of MPI::Reduce with function to integrate
// ==================================================================
#include <unistd.h>
#include <iostream>
#include <cstdlib>
#include <unistd.h> // for sleep
#include <sstream>
using namespace std;
#include "ezmpi.h"
using namespace ez::mpi;
/**
* function to integrate f(x)
*/
double f(double x) {
return x * x;
}
/**
* integrate from
* @param a lower bound
* @param b upper bound
* @param n number of steps
*/
double integrate(double a, double b, int n) {
double dx = (b-a) / n;
double sum = 0;
for (int i=1; i<n; ++i) {
sum += f(a + i * dx);
}
return sum * dx;
}
/**
* run master and slaves
*/
void run(int argc, char *argv[]) {
Process p(argc, argv);
// data to send
double a = 1.0;
double b = 3.0;
int steps = 10000000;
double x_range = (b - a) / p.max();
int steps_range = steps / p.max();
double local_a = a + p.id() * x_range;
double local_b = local_a + x_range;
double local_result = integrate(local_a, local_b, steps_range);
p << "integrate from " << local_a << " to " << local_b;
p << " during " << steps_range << " steps" << endl;
p << "local_result=" << local_result << endl;
double final_result = 0;
p.synchronize();
p.reduce(local_result, final_result, MPI::SUM);
// expected result should be (9 - 1/3) = 8.6666
if (p.is_master()) {
p << "final result=" << final_result << endl;
}
sleep(1);
p.logs(cout);
}
/**
* main function
*/
int main(int argc, char ** argv) {
// call a function that contains code for master and slave
// in order to avoid problems of initialization and finalization
run(argc, argv);
exit(EXIT_SUCCESS);
}
On calcule l'intégrale de la fonction $f(x) = x^2$ entre $x=1$ et $x=3$. Pour cela on utilise la méthode des rectangles.
On veut 10_000_000 de rectangles entre 1 et 3. Si on utilise 4 processeurs on aura donc $10\_000\_000 / 4 = 2\_500\_000$
itérations par processeur. Au final, le résultat de la somme de chaque partie de l'intégrale est renvoyé au maître
qui en fait la somme.
- le processeur 0 intègre entre 1 et 1.5 avec une intégrale de 0.79
- le processeur 1 intègre entre 1.5 et 2 avec une intégrale de 1.54
- le processeur 2 intègre entre 2 et 2.5 avec une intégrale de 2.54
- le processeur 3 intègre entre 2.5 et 3 avec une intégrale de 3.79
---------------------
CPU 0
---------------------
2016-08-24.02:37:35 cpu 0/4: pid=22172, rank=0
2016-08-24.02:37:35 cpu 0/4: integrate from 1 to 1.5 during 2500000 steps
2016-08-24.02:37:35 cpu 0/4: local_result=0.791666
2016-08-24.02:37:35 cpu 0/4: reduce gives value=8.66666
2016-08-24.02:37:35 cpu 0/4: final result=8.66666
---------------------
CPU 1
---------------------
2016-08-24.02:37:35 cpu 1/4: pid=22173, rank=1
2016-08-24.02:37:35 cpu 1/4: integrate from 1.5 to 2 during 2500000 steps
2016-08-24.02:37:35 cpu 1/4: local_result=1.54167
2016-08-24.02:37:35 cpu 1/4: reduce gives value=0
---------------------
CPU 2
---------------------
2016-08-24.02:37:35 cpu 2/4: pid=22174, rank=2
2016-08-24.02:37:35 cpu 2/4: integrate from 2 to 2.5 during 2500000 steps
2016-08-24.02:37:35 cpu 2/4: local_result=2.54167
2016-08-24.02:37:35 cpu 2/4: reduce gives value=0
---------------------
CPU 3
---------------------
2016-08-24.02:37:35 cpu 3/4: pid=22175, rank=3
2016-08-24.02:37:35 cpu 3/4: integrate from 2.5 to 3 during 2500000 steps
2016-08-24.02:37:35 cpu 3/4: local_result=3.79167
2016-08-24.02:37:35 cpu 3/4: reduce gives value=0
5.8. Gather et scatter
MPI propose deux opérations inverses :
- scatter (split) qui permet de décomposer un tableau en différentes parties égales et de les envoyer aux autres processus
- gather (join) qui exécute l'opération inverse : à partir de tableaux locaux détenus par chacun des processus, on crée un tableau
global plus grand qui est la concaténation des tableaux locaux
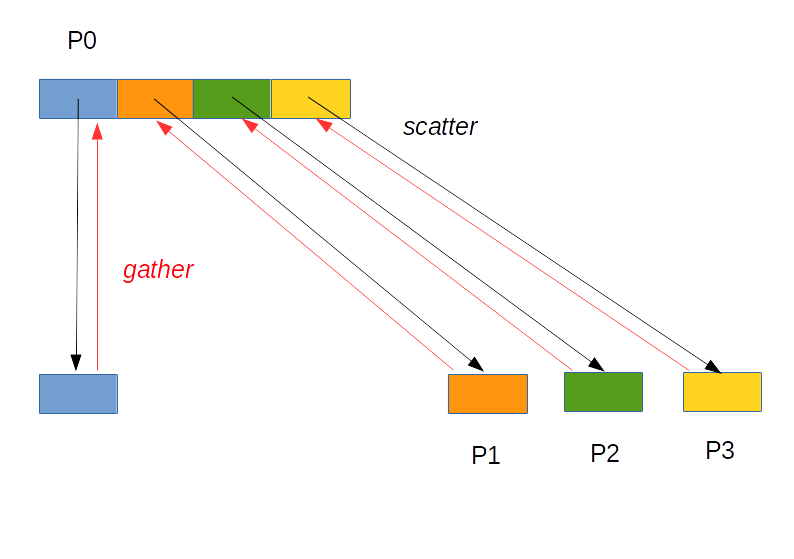
Voici un exemple pour lequel $K$ processus créent des tableaux de 10 entiers qui sont envoyés au master (processus de rang 0) :
- Processus 0 : [1, 2, ... , 10]
- Processus 1 : [11, 12, ..., 20]
- Processus $i$ : [$(i*10+1)$, ...,$(i+1)*10$ ]
// ==================================================================
// Author: Jean-Michel Richer
// Date: 20 Aug 2016
// Purpose: Use of MPI::Scatter
// The master process creates its own local data and they are send
// to other process (of rank not equal to 0)
// ==================================================================
#include <unistd.h>
#include <iostream>
#include <cstdlib>
#include <unistd.h> // for sleep
using namespace std;
#include "ezmpi.h"
using namespace ez::mpi;
/**
* run master and slaves
*/
void run(int argc, char *argv[]) {
Process p(argc, argv);
const int local_data_size = 10;
int *local_data;
int global_data_size = p.max() * local_data_size;
int *global_data = NULL;
local_data = new int [ local_data_size ];
for (int i=0; i<local_data_size; ++i) {
local_data[i] = 0;
}
if (p.is_master()) {
// master process will send data to others
global_data = new int [ global_data_size ];
for (int i=0; i<global_data_size; ++i) global_data[i] = i+1;
p << "global array = [";
for (int i=0; i<global_data_size; ++i) p << global_data[i] << " ";
p << "]" << endl;
}
// Master sends data to all others
p.scatter(global_data, local_data_size, local_data);
// Each process reports the data it has received
p << "local array=[";
for (int i=0; i<local_data_size; ++i) {
p << local_data[i] << " ";
}
p << "]" << endl;
sleep(1);
p.logs(cout);
}
// ==================================================================
// C++ version
// ==================================================================
int main(int argc, char ** argv) {
// call a function that contains code for master and slave
// in order to avoid problems of initialization and finalization
run(argc, argv);
exit(EXIT_SUCCESS);
}
\$ mpirun -n 4 ./ezmpi_scatter.exe
====================
=== FINAL RESULT ===
====================
---------------------
CPU 0
---------------------
14:15:05 cpu 0/4: global array = [1 2 3 4 5 6 7 8 9 10 11 12 13 14 15 16 17 18 19 20 21 22 23 24 25 26 27 28 29 30 31 32 33 34 35 36 37 38 39 40 ]
14:15:05 cpu 0/4: scatter
14:15:05 cpu 0/4: local array=[1 2 3 4 5 6 7 8 9 10 ]
---------------------
CPU 1
---------------------
14:15:05 cpu 1/4: scatter
14:15:05 cpu 1/4: local array=[11 12 13 14 15 16 17 18 19 20 ]
---------------------
CPU 2
---------------------
14:15:05 cpu 2/4: scatter
14:15:05 cpu 2/4: local array=[21 22 23 24 25 26 27 28 29 30 ]
---------------------
CPU 3
---------------------
14:15:05 cpu 3/4: scatter
14:15:05 cpu 3/4: local array=[31 32 33 34 35 36 37 38 39 40 ]
// ==================================================================
// Author: Jean-Michel Richer
// Date: Aug 2020
// Last modified: November 2020
// Purpose: Use of MPI::Gather
// Each process creates its own local data and they are all send
// to the master process (of rank 0)
// ==================================================================
#include <unistd.h>
#include <iostream>
#include <cstdlib>
#include <unistd.h> // for sleep
#include <sstream>
using namespace std;
#include "ezmpi.h"
using namespace ez::mpi;
/**
* run master and slaves
*/
void run(int argc, char *argv[]) {
// data to send
float *data;
int data_size;
// Create Process from ezmpi
// that retrives cpu rank (id), # cpus (max), cpu name (name)
Process p(argc, argv);
const int local_data_size = 10;
int *local_data;
int global_data_size = 0;
int *global_data = NULL;
// each processor creates its local data
local_data = new int [ local_data_size ];
for (int i=0; i<local_data_size; ++i) {
local_data[i] = (p.id() * 10) + i + 1;
}
p << "local array=[";
for (int i=0; i<local_data_size; ++i) {
p << local_data[i] << " ";
}
p << "]" << endl;
if (p.is_master()) {
// master process will gather data from others
global_data_size = p.max() * local_data_size ;
global_data = new int [ global_data_size ];
for (int i=0; i<global_data_size; ++i) global_data[i] = 0;
}
p.gather(local_data, local_data_size, global_data);
if (p.is_master()) {
p << "global array = [";
for (int i=0; i<global_data_size; ++i) {
p << global_data[i] << " ";
}
p << "]" << endl;;
}
sleep(1);
p.logs(cout);
}
// ==================================================================
// C++ version
// ==================================================================
int main(int argc, char ** argv) {
// call a function that contains code for master and slave
// in order to avoid problems of initialization and finalization
run(argc, argv);
exit(EXIT_SUCCESS);
}
\$ mpirun -n 4 ./ezmpi_gather.exe
====================
=== FINAL RESULT ===
====================
---------------------
CPU 0
---------------------
14:19:02 cpu 0/4: local array=[1 2 3 4 5 6 7 8 9 10 ]
14:19:02 cpu 0/4: gather
14:19:02 cpu 0/4: global array = [1 2 3 4 5 6 7 8 9 10 11 12 13 14 15 16 17 18 19 20 21 22 23 24 25 26 27 28 29 30 31 32 33 34 35 36 37 38 39 40 ]
---------------------
CPU 1
---------------------
14:19:02 cpu 1/4: local array=[11 12 13 14 15 16 17 18 19 20 ]
14:19:02 cpu 1/4: gather
---------------------
CPU 2
---------------------
14:19:02 cpu 2/4: local array=[21 22 23 24 25 26 27 28 29 30 ]
14:19:02 cpu 2/4: gather
---------------------
CPU 3
---------------------
14:19:02 cpu 3/4: local array=[31 32 33 34 35 36 37 38 39 40 ]
14:19:02 cpu 3/4: gather
5.9. Broadcast (diffuser)
Enfin, l'opération broadcast envoie à tous les esclaves une copie d'un tableau
détenu par le maître par exemple :
// ==================================================================
// Author: Jean-Michel Richer
// Date: Aug 2020
// Last modified: November 2020
// Purpose: Use of MPI::BCast
// The master process creates its own local data and they are send
// to other process (of rank not equal to 0)
// ==================================================================
#include <unistd.h>
#include <iostream>
#include <cstdlib>
#include <unistd.h> // for sleep
using namespace std;
#include "ezmpi.h"
using namespace ez::mpi;
/**
* run master and slaves
*/
void run(int argc, char *argv[]) {
Process p(argc, argv);
int global_data_size = 100;
int *global_data = NULL;
global_data = new int [ global_data_size ];
for (int i=0; i<global_data_size; ++i) {
global_data[i] = 0;
}
if (p.is_master()) {
// master process will send data to others
for (int i=0; i<global_data_size; ++i) global_data[i] = i+1;
p << "global array = [";
for (int i=0; i<global_data_size; ++i) p << global_data[i] << " ";
p << "]" << endl;
}
// Master sends data to all others
//p.synchronize();
p.broadcast(global_data, global_data_size, 0);
// Each process reports the data it has received
p << "local copy array=[";
for (int i=0; i<global_data_size; ++i) {
p << global_data[i] << " ";
}
p << "]" << endl;
sleep(1);
p.logs(cout);
}
// ==================================================================
// C++ version
// ==================================================================
int main(int argc, char ** argv) {
// call a function that contains code for master and slave
// in order to avoid problems of initialization and finalization
run(argc, argv);
exit(EXIT_SUCCESS);
}
\$ mpirun -n 4 ./ezmpi_broadcast.exe
====================
=== FINAL RESULT ===
====================
---------------------
CPU 0
---------------------
17:08:27 cpu 0/4: global array on master = [1 2 3 4 5 6 7 8 9 10 ]
17:08:27 cpu 0/4: broadcast
17:08:27 cpu 0/4: global array (after broadcast) =[1 2 3 4 5 6 7 8 9 10 ]
---------------------
CPU 1
---------------------
17:08:27 cpu 1/4: global array on slave = [0 0 0 0 0 0 0 0 0 0 ]
17:08:27 cpu 1/4: broadcast
17:08:27 cpu 1/4: global array (after broadcast) =[1 2 3 4 5 6 7 8 9 10 ]
---------------------
CPU 2
---------------------
17:08:27 cpu 2/4: global array on slave = [0 0 0 0 0 0 0 0 0 0 ]
17:08:27 cpu 2/4: broadcast
17:08:27 cpu 2/4: global array (after broadcast) =[1 2 3 4 5 6 7 8 9 10 ]
---------------------
CPU 3
---------------------
17:08:27 cpu 3/4: global array on slave = [0 0 0 0 0 0 0 0 0 0 ]
17:08:27 cpu 3/4: broadcast
17:08:27 cpu 3/4: global array (after broadcast) =[1 2 3 4 5 6 7 8 9 10 ]
5.10. Liens
5.11. Exercices
Exercice 5.1
Une suite de Syracuse est une suite d'entiers naturels définie de la manière suivante : on part d'un nombre entier strictement positif
- s'il est pair, on le divise par 2
- s'il est impair, on le multiplie par 3 et on ajoute 1
Cette suite possède la propriété de converger vers 1 après un certain nombre d'étapes.
Mettre en place une solution MPI avec trois instances :
- le maître génére un nombre entier aléatoire $x$
- si le nombre $x$ est pair, il l'envoie à l'esclave n°1 qui retourne $x/2$
- si le nombre $x$ est impair, il l'envoie à l'esclave n°2 qui retourne $3x+1$
- à chaque étape, le maître affiche la nouvelle valeur de $x$
Le maître comptera le nombre d'appels à l'esclave n°1 et l'esclave n°2.
Lorsque le maître recoît la valeur 1, il s'arrête et envoie un code d'arrêt aux esclaves (un nombre négatif par exemple).
Puis il affiche le nombre d'appels à chaque esclave.
Exercice 5.2
Objectif : Écrire un programme MPI pour rechercher un élément dans un tableau.
Description :
- créer 5 processus
- générer un tableau d'un million d'entiers aléatoires sur le processus maître (processus de rang 0)
- répartir le tableau entre les différents processus esclaves en utilisant la fonction MPI_Scatter
- le maître génère un nombre aléatoire $x$ et demande à chaque esclave de rechercher le nombre d'occurrences de $x$ dans son tableau et de retourner le résultat
- afficher sur le maître, le nombre d'occurrences par esclave